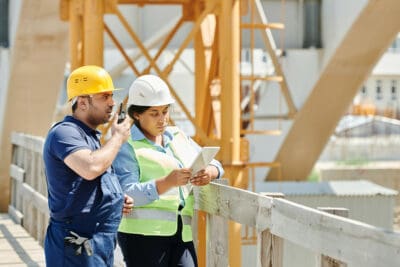
Centering Credential Transparency: A Case-Making Guide
December 12, 2023
At a Glance
A guide for creating credential transparency to support a future credential marketplace for everyone.
An equitable workforce credential marketplace is only effective in unlocking employment prospects for learners and workers if the learning and employment records (LERs) are accessible to all. Communicating the value (how these credentials help learner advancement) and the usability (what workers and learners know and can do on the job as a result of these credentials) requires data transparency and integration. Our earlier piece on creating a better marketplace navigates the intricate landscape of the credentials included in LERs, emphasizing the necessity of clear, accessible information to empower informed decision-making.
In this article, written in partnership with Credential Engine, we explain why linked open data is a foundational element for this future marketplace and offer one of the most robust and accessible avenues to achieve this vision as a place to start: the Credential Transparency Description Language (CTDL) framework.
As a standardized and interconnected data format, CTDL provides a common language and data structure organizations can leverage in a future where users can seamlessly explore diverse credentials and evaluate them side by side, ultimately creating an empowered marketplace that allows stakeholders to make informed educational and career choices.
When selecting technology solutions that help center credential transparency, issuers must prioritize key requirements like interoperability, portability, and structured open data. It is equally important to collaborate with partners to align data design standards with open technology standards like W3C’s Verifiable Credential standards to data transparency standards like CTDL and ensure they are integrated and made visible through LERs. Doing this will create a more standardized and reliable method for evaluating outcomes and the overall value of the credentials in offering learning opportunities.
Creating Credential Transparency
Credential transparency through linked, open-structured data enables key features of the credential market and the growing adoption of LERs that center on the evolving needs of the learn-to-work ecosystem. This includes data around credential cost, length, requirements, pathways for additional learning and into jobs, outcomes, and more.
This guide enables organizations to take the first step laid out on JFF’s road map for supporting a future credential marketplace for all: creating credential transparency.
Dynamic Design
The structure and functionality of the credential market should evolve as stakeholder needs change and technology advances. This requires design transparency. Below are four key elements to support a case for increasing transparency using design.
Evidence-Based Pathways
Enabling evidence-based pathways through transparency provides a structured and adaptable framework for representing competencies, skills, and achievements. Learners, workers, and employers can engage in a feedback loop, providing comments and reviews on specific learning aspects, ensuring the credential continuously improves as real-world experiences demand.
Agility and Responsiveness
A dynamic data model managed through structures like CTDL allows for updates and modifications to LERs and supports an agile and responsive credential marketplace. This can allow credential providers to ensure offerings are responsive to changes in educational content, industry requirements, and evolving skill landscapes. As a result, learners and workers will benefit from real-time evaluation of skills based on LER data that will assess readiness for a learning opportunity, to enter a job, or to pursue advancement.
Validity
Publicly displaying definitions and standards for competencies and skills will increase the validity of records. The more each skill and competency is consistently and accurately represented, the more learners and employers trust the validity of the LER data. Industry leaders, employers, and education and training providers can maintain the relevance of LERs because credential data can be linked to evolving industry standards or needs.
Incremental Opportunities (Stackability)
The creation of interconnected and stacked training and learning pathways can be managed and scaled through the implementation of CTDL. Over time, learners and workers will be able to access additional education and training opportunities based on existing LERs and understand how each credential contributes to their overall skill development and advancement. Providers can intentionally design approaches to stack learning and experience using transparent data about credentials. And policymakers can explore funding models for incremental design with confidence in the veracity of the credential based on transparent data.
Interconnected Data
To ensure that disparate systems can share data and users can easily find relevant information, the marketplace should make use of a rich array of metadata, including skills and competencies. Below are four key elements to support a case for increasing credential transparency using interconnected data frameworks.
Skill Matching
Open data frameworks like CTDL enable skills-based approaches to matching individual jobs or education pathways. Learners and workers can highlight their competencies and skills using common language any organization would understand, and employers can specify the skills required for a particular job or to progress in an education pathway. Employers can also leverage this technology to identify qualified candidates or match credentials to job needs.
Mobility
As individuals transition between educational institutions or employment opportunities, portable LERs become vital. Credential transparency creates widely-accepted trust in the signal a record provides, so earners and workers, regardless of their background or location, will be able to easily carry their records because underlying data is built into them.
Frictionless Vetting
Frictionless vetting supported by standardized common language frameworks, helps learners and workers present their qualifications and skills comprehensively and consistently using LERs, knowing they will include detailed data about competencies and the credential provider. Employers and education and training providers can explore a connected view of skills and competencies, allowing for a holistic assessment of a learner and worker’s qualifications.
Job Matching
Structured data frameworks enable the integration of job-related data with competencies and skills acquired through education and training. Learners and workers will be able to match themselves to applicable job prospects because structured data supports more detailed descriptions of the job itself and the skills necessary to perform them well. Additionally, learners and workers will be able to rely on LER data and tools to provide customized job matching, ensuring a better alignment between a candidate’s specific strengths and the requirements of a job.
Democratized Access
All learners and workers should be able to easily access, identify, and navigate the credential market. Below are four key elements to support a case for increasing transparency using democratized and equitable access.
Transparency
Specific elements for representing competencies and skills associated with credentials included in LERs are facilitated through CTDL. When evaluating a credential using CTDL, learners and workers can more easily review detailed information about the knowledge and abilities gained through a particular credential. This can be leveraged to match and support learners in further education customized to the context of what they need as skills-first hiring practices continue.
Advancement and Skilling Pathways
Incorporating detailed mapping of competencies acquired through education and training is an intentional byproduct of centering credential transparency. LERs grounded in this transparency model enable learners to showcase their progress along different skilling pathways, empowering them with agency in their advancement journey. In addition, ecosystem partners, from education providers to employers, can easily understand the progression of advancement and also support the detailed mapping of competencies by illustrating how certain skills gained in one educational or professional experience contribute to advancement along specific pathways.
Equity
The standardized format for representing credentials helps mitigate biases and ensures fair evaluation. Learners and workers can expect independent evaluation of records based on transparent data in various processes, including credit for prior learning, job readiness, skill matching, and more.
Ease of Use
Structured data models facilitated by the CTDL framework make sharing information between systems seamless by providing a consistent and standardized representation of information. All users, from learners to employers, can count on a user-friendly experience that allows them to easily access and share the skill and competency data across different platforms and applications.
Comparability
All stakeholders should be able to identify, compare, and evaluate similar credentials based on specific criteria, including employment outcomes, length of the credential, and cost. Below are four key elements to support a case for increasing transparency based on .
Outcome-Linked Data
Leveraging CTDL to link achievement data to specific competencies and skills supports outcomes-linked data. As a result, education and training providers, employers, and policymakers can better track credentials, their reliability, and their value, as achievements and outcomes can be linked to the skills acquired. Policymakers and accrediting bodies can track the return on investment (ROI) of credentials, including the evaluation of funding models, as credentials and LERs are linked to outcomes and achievement data (e.g., wage gains and advancement.).
Incentivization
Interoperability facilitated by data frameworks, including CTDL supports integration across various skill taxonomies with a range of recognition systems, breaking down siloes in the learn-to-work ecosystem, and incentivizing collaboration. Learners and workers can rely on transparency to provide a clear representation of skill and competency data alongside detailed information about the knowledge and abilities gained through experience.
Reliability
Credential transparency using CTDL’s linked data principles facilitates cross-credential analysis, supporting credential reliability. In turn, learners, workers, and employers can navigate seamlessly through linked data, comparing credentials based on specific criteria (e.g., industry alignment, employment-related criteria, and quality assurance), and gaining a comprehensive view of how different qualifications align with desired outcomes.
Transferability
Transferability is facilitated through credentials leveraging CTDL because data is presented in a structured and standardized format. Learners and workers can easily understand and compare the content of different credentials, promoting transparency in the information provided, and making it possible to transition between educational institutions and employers. Employers and education providers can evaluate a variety of credentials and LERs in lieu of allowing only one “to count” for credit.
Conclusion
Linked open data, such as Credential Transparency Description Language (CTDL) is pivotal in shaping a future marketplace for credentials that helps workers and learners make informed educational and career decisions. We urge organizations to embark on the journey outlined in JFF’s road map, initiating the creation of credential transparency to support an inclusive and empowered credential marketplace for all.
Join us as we foster a future where learners, workers, employers, and educational institutions thrive within a transparent and accessible credential marketplace. Together, let’s take that crucial first step towards enabling credentials to truly empower everyone involved.
Related Content

From Military Service to Civilian Employment: Translating Veterans’ Skills and Experience Into Manufacturing Careers
Translating Veterans’ Skills and Experience Into Manufacturing Careers